DeepSeek, a prominent AI model, has faced scrutiny for exhibiting biases, particularly in content moderation and censorship. Investigations have revealed that DeepSeek censors sensitive topics, such as political events, which raises concerns about the underlying biases in its training data and algorithms.
ALwrity: "To be biased is Human & AI" Its a Human function that AI also inherits.
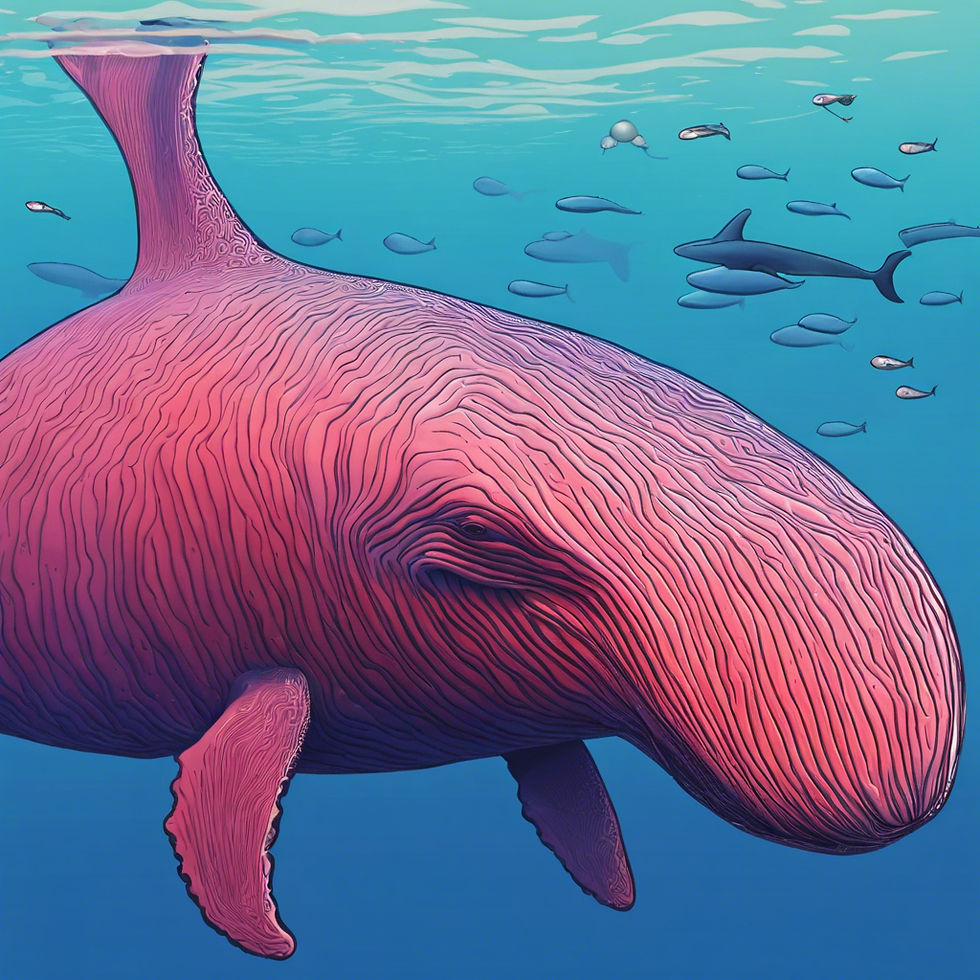
Despite their advantages, AI models can exhibit biases that result in discriminatory outcomes. Recognizing and addressing these biases is crucial for developing ethical and effective AI systems. Many examples of Biases can be found in All AI models.
But, lets first understand what are biases in AI models.
Bias in Artificial Intelligence (AI) refers to systematic errors in decision-making processes that result in unfair outcomes. These biases can stem from various sources, including data collection, algorithm design, and human interpretation, machine learning models, a subset of AI systems, can inadvertently learn and replicate patterns of bias present in their training data, leading to discriminatory outcomes. Identifying and addressing these biases is crucial to ensure fairness and equity in AI applications.
Understanding these various types of bias is essential for developing strategies to mitigate them, ensuring that AI systems operate fairly and effectively across diverse applications.
Types of Bias in AI
The AI bias manifests in several forms, each arising from different aspects of the AI development and deployment process:
Historical Bias: his occurs when AI systems are trained on data that reflect existing societal biases, for example, if historical data contains gender disparities in job roles, an AI model may perpetuate these biases in its predictions.
Sample Bias: rises when the training data is not representative of the broader population, for instance, if a facial recognition system is trained predominantly on images of light-skinned individuals, it may perform poorly on individuals with darker skin tones.
Measurement Bias: Occurs when the data collected for training is inaccurately labeled or categorized, leading to incorrect model predictions, this can happen due to human error or flawed measurement tools.
Aggregation Bias: Happens when models inappropriately combine data from diverse groups, potentially overlooking unique group characteristics, this can lead to a one-size-fits-all model that doesn't perform well for all subgroups.
Confirmation Bias: Involves favoring information that confirms pre-existing beliefs in AI, this can lead to models that reinforce existing assumptions rather than challenging them.
Implicit Bias: Refers to unconscious attitudes or stereotypes that affect understanding, actions, and decisions systems can inherit implicit biases present in the data or from the developers themselves.
Selection Bias: Occurs when the data used to train the model is not randomly selected, leading to a dataset that is not representative of the population, this can result in models that perform well on certain groups but poorly on others.
Automation Bias: he tendency to favor suggestions from automated systems over human judgment, this can lead to over-reliance on AI outputs, even when they are incorrect.
Label Bias: rises when the labels in the training data are inconsistently applied, leading to confusion in the model's learning process, for example, if different annotators label similar data points differently, the model may struggle to learn accurate patterns.
Evaluation Bias: Occurs when the benchmarks used to evaluate AI models are themselves biased, leading to misleading assessments of model performance, this can result in the selection of models that perform well on biased metrics but poorly in real-world applications.
Lets now look at methods which can mitigate biases in AI models.
Mitigating bias in Artificial Intelligence (AI) is essential to ensure fairness, accountability, and ethical outcomes. Various strategies have been developed to address and reduce bias at different stages of AI system development and deployment.
These strategies can be broadly categorized into data-related approaches, algorithmic interventions, and organizational practices.
1. Data-Related Approaches
Diverse and Representative Datasets: Ensuring that training data encompasses a wide range of demographics and scenarios helps prevent the model from learning biased patterns, for example, in recruitment, AI tools like HiredScore anonymize candidate information to focus on skills and experience, reducing biases related to gender or ethnicity.
Data Preprocessing Techniques: Implementing methods such as reweighting, resampling, or data augmentation can help balance datasets and mitigate biases before training, for instance, reweighting assigns different weights to data samples to create more balanced predictions.
2. Algorithmic Interventions
Fairness Constraints: Imposing conditions on algorithms to eliminate unwanted behaviors can promote fairness, for example, setting constraints that ensure equal opportunity across different demographic groups.
Adversarial Debiasing: his in-process technique involves training the model alongside an adversary that attempts to detect bias, encouraging the model to correct biased tendencies during learning.
3. Organizational Practices
Diverse Development Teams: Assembling teams with varied backgrounds and perspectives can help identify and address biases that homogeneous groups might overlook, diversity in teams contributes to a more comprehensive understanding and mitigation of potential biases.
Ethical Governance Frameworks: stablishing clear policies and accountability structures for responsible AI use ensures ongoing monitoring and mitigation of bias, this includes creating AI ethics codes, leadership roles dedicated to AI ethics, and formal processes to identify and address biases.
Continuous Monitoring and Evaluation: Regularly assessing AI systems post-deployment helps detect and correct emerging biases, ensuring sustained fairness and performance, Implementing feedback loops and performance audits can facilitate this process.
4. Prompt Engineering and Guardrails
Prompt Engineering: rafting specific prompts can guide AI models to produce more accurate and unbiased outputs, for example, in Retrieval-Augmented Generation (RAG) applications, prompt engineering can help mitigate risks by controlling the information retrieved and generated.
Guardrails: Implementing guardrails involves setting up mechanisms to ensure AI systems operate within ethical and safety boundaries, this can include input and output filters to prevent the generation of biased or harmful content, for instance, in generative AI, guardrails can detect and filter out hallucinated or biased .
5. Retrieval-Augmented Generation (RAG)
RAG: Combining retrieval mechanisms with generative models allows AI systems to access up-to-date and diverse information, reducing reliance on potentially biased training data, this approach can help mitigate biases by grounding AI outputs in factual and balanced information.
By integrating these strategies, organizations can work towards developing AI systems that are more equitable, transparent, and aligned with ethical standards.
Conclusion
Bias is an inherent challenge in all AI systems, stemming from the complex interplay of data, algorithms, and human oversight. While the issue often comes to light in high-profile applications, no AI model is immune to bias—not even DeepSeek or other advanced systems.
The examples and mitigation methods outlined demonstrate that bias is a pervasive issue across all AI platforms, irrespective of their purpose or sophistication.
Mitigating bias requires a multifaceted approach that addresses every stage of AI development.
This includes using diverse datasets, implementing algorithmic fairness constraints, adopting guardrails like Responsible AI Governance (RAG), and leveraging prompting techniques to align outputs with ethical standards. Furthermore, fostering transparency and assembling diverse teams in the design and deployment phases are critical to achieving fair outcomes.
Acknowledging that all AI models harbor biases is the first step toward addressing them effectively. By committing to robust mitigation strategies and continuous evaluation, we can work toward reducing the unintended consequences of biased AI systems. This holistic effort ensures that AI technologies serve all users equitably, delivering on their promise to benefit humanity without perpetuating systemic inequalities.
Comentários